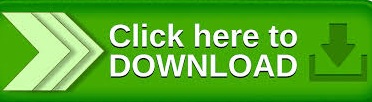
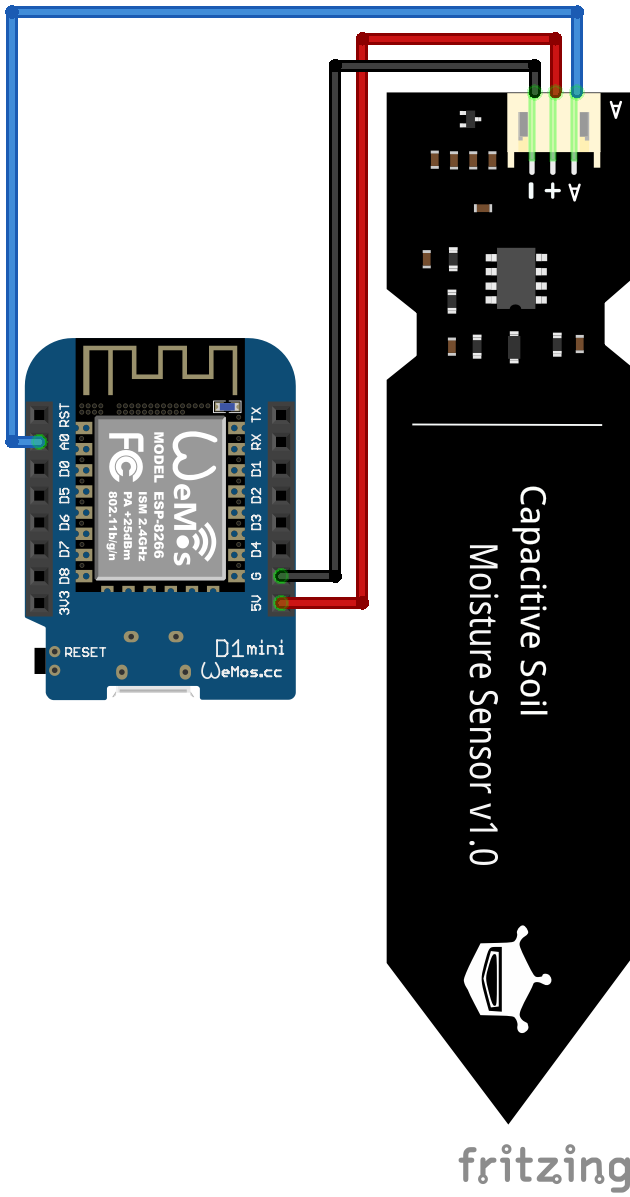
Vehicular edge computing (VEC) is a new computing paradigm with great potential of enhancing SCADA system performance by offloading applications from the resource-constrained SCADA system to lightweight and ubiquitous VEC servers. The emergence of computation intensive and delay sensitive applications makes it quite a challenge for IoT devices in SCADA systems with very weak computing capacity. The simulation results show that the performance of the CBLA algorithm outperforms two peer algorithms in terms of the number of barrier paths and the average number of nodes in each barrier path.Ībstract: In commercial buildings, about 40%–50% of the total electricity consumption is attributed to Heating, Ventilation, and Air Conditioning (HVAC) systems, which places an economic burden on building operators In this paper, we intend to minimize the energy cost of an HVAC system in a multi-zone commercial building with the consideration of random zone occupancy, thermal comfort, and indoor air quality comfort Due to the existence of unknown thermal dynamics models, parameter uncertainties (eg, outdoor temperature, electricity price, and number of occupants), spatially and temporally coupled constraints associated with indoor temperature and CO2 concentration, a large discrete solution space, and a non-convex and non-separable objective function, it is very challenging to achieve the above aim To this end, the above energy cost minimization problem is reformulated as a Markov game Then, an HVAC control algorithm is proposed to solve the Markov game based on multi-agent deep reinforcement learning with attention mechanism The proposed algorithm does not require any prior knowledge of uncertain parameters and can operate without knowing building thermal dynamics models Simulation results based on real-world traces show the effectiveness, robustness and scalability of the proposed algorithmĪbstract: With the development of microprocessor technology, massive IoT devices are deployed in smart cities which promotes numerous supervisory control and data acquisition (SCADA) systems to accomplish the key issue.

CBLA selects an optimal IoT node to construct the barrier path by learning. Each IoT node equips a learning automaton. The CBLA includes four crucial phases which are initialization phase, learning phase, monitoring phase, and repairing phase. We propose a distributed CIC barrier path (CICBP) construction approach based on learning automata (CBLA). To overcome the disadvantages in the existing works on barrier coverage, we adopt a novel and widely adopted confident information coverage (CIC) model as the fundamental coverage model and formulate the CIC barrier path construction (CICBC) problem with the goals of maximizing the number of barrier paths and minimizing the amount of IoT nodes in each barrier path.
#Otomata soil sensors how to
Aiming to provide coverage service for ocean border environmental surveillance, this article studies the barrier coverage problem which investigates how to select a collection of IoT nodes to obtain an IoT node chain and build barrier paths to detect intruders and trespassers crossing the border region of interest. With the merits of intelligent capacity, desirable scalability, and high reliability, the IoT recently has been applied for smart ocean applications to provide protection for ocean environment monitoring and surveillance. Abstract: As an emerging network paradigm, the Internet of Things (IoT) which consists of a significant number of multifunctional and heterogeneous IoT nodes has attracted dramatic attentions from both academia and industry.
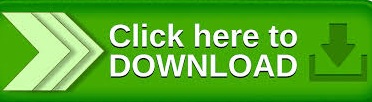